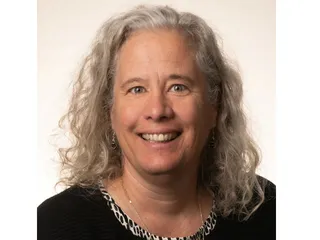
Analytics for emerging enterprise risks
In the early years of enterprise risk management (ERM), consultants took varying approaches to the implementation of a firm’s enterprise risk framework. Many of the approaches had three distinct phases in common: risk qualification, risk quantification, and risk strategy development.
“The core processes of ERM provide captives with a reliable framework on an initial and an ongoing basis.”
The first phase focused on identifying the firm’s key risks and determining qualitative assessments of their frequency and severity. The second phase moved to an in-depth analysis and quantification of these risks, often involving statistical modelling and confidence level analyses. The strategy and implementation phase focused on new, alternative ways of mitigating and financing risks, often seeking to combine them into a single portfolio.
Prior to the introduction of ERM, many of these risks had never been specifically addressed, or even reviewed. While the types of risks identified in this process continue to shift and expand, the framework for identifying and assessing them still relies on the same three phases. More than ever, though, firms are choosing to finance enterprise risks by placing them into captive insurance companies.
The steps for placing these risks into a captive parallel the three phases. After a risk is identified, quantified, and indicated to be appropriate for the captive, the programme is structured to incorporate the risk. The quantification stage occurs both before the risk is placed in the captive (loss projections and premium calculations) and after the risk is placed into the captive (ongoing loss projections and reserve analyses).
Creating the pre-captive loss projections will likely be an iterative process, as multiple loss scenarios with specific policy types (claims-made vs occurrence) and retention assumptions are often considered. Once created, though, these loss projections serve as analytic guidance, informing the programme structure for the risk and ultimately serving as the basis for post-formation analysis.
Initial data considerations
The data available when completing the initial loss projections may be limited or difficult to obtain. When collecting data for new or emerging risks, the following suggestions may be helpful:
- Collect any internal loss data that has been maintained for a risk. For example, if the risk is “loss of key customer”, what internal records show that loss history over the last several years? Even if very limited, this data can be useful in determining appropriate frequency assumptions. For previously insured risks, loss runs are sufficient.
- Review the risk for appropriate exposure base information. Using cyber risk as an example, is data maintained regarding the number of electronic transactions? Similarly, for warranty risks, is the amount of product sold or manufactured by year available?
- Gather any quotes that have been obtained for transferring the risk in the insurance market. A market quote for a similar programme structure can provide significant support for additional analytics.
- Obtain qualitative input from management and key personnel on the anticipated frequency and severity of the risk, based on internal observations as well as peer discussions. This might be accomplished informally via discussion or through a brief questionnaire.
- Discuss what appropriate industry benchmark data might be available to supplement the actual data. For example, if parametric wind is being assessed, historical windspeed data for the region should be available.
Initial analytical considerations
If the data is credible, standard actuarial approaches (eg, development methods or loss ratio analytics) may prove to be reasonable. However, risks of this nature are often low in frequency and potentially high in severity, requiring non-traditional analytical approaches, such as simulations using frequency and severity distributions.
When preparing initial loss projections for risks with limited data or low frequency, the following should be considered to help determine methodology:
- Frequency and severity parameters may be estimated based on unique data and qualitative information, then supplemented with industry information to simulate aggregate losses at various retentions;
- Actual premium information for historical years may be adjusted to appropriate retention levels and policy coverages as available. From there, expected loss ratios can be used to estimate projected losses; and
- If market quotes are available for the projected period, these may also be relied upon with the use of a loss ratio method.
Due to the low-frequency and potential high-severity nature of many of these risks, aggregated losses at higher confidence levels may be reviewed. Results from this review may be incorporated into proforma financial statements. This can highlight the potential financial impact on the captive due to adverse losses during specific time periods.
Ongoing data and analytical considerations
As the captive programme moves forward, the loss projection analysis will almost certainly require annual updates. Since actual, capturable data will become available for the included risks over time, it should be collected and used to refine both parameter selection and available methods. A reserve analysis will also be needed on a yearly basis (at minimum) to review the historical losses and determine the amount of outstanding liabilities for the captive’s balance sheet.
This analysis is completed as of a specific accounting date, and only losses that have occurred prior to the evaluation date are included. The required reserves (or outstanding liabilities) comprise both the case reserves and incurred but not reported losses (IBNR). Simply put, the loss projections are future estimates used for premium and accrual purposes, and the reserve analyses estimate the historical periods’ ultimate outstanding losses for balance sheet purposes.
For risks with credible data or a longer tail, the ultimate losses are typically determined with consideration to the actual loss data as it emerges. Many enterprise risks, however, are short-tailed in nature because they are written either on a claims-made basis or are on an occurrence basis with an inherently short tail.
For example, the loss of a key employee is a naturally short-tailed risk, irrespective of the policy trigger structure. Since the claim is triggered by a clear and known separation date with HR, knowledge of this event’s occurrence is nearly immediate. In this case, projections may require more advanced simulation techniques, but the analysis of reserves for the risk will likely be straightforward due to a lack of IBNR. Similarly, claims-made policies with no reported claims may not require IBNR if no pipeline claims are confirmed.
The core processes of ERM provide captives with a reliable framework on an initial and an ongoing basis: identify, quantify, and mitigate/finance risks. This framework assumes the analytical process for captives to be continuous and evolving, as new risks will be evaluated for potential inclusion and current risk profiles will change over time. As a captive matures, advanced correlation analytics and financial statement modelling may be necessary to ensure the most efficient strategies and programmes are in place.
Risks and risk portfolios in the insurance landscape are constantly evolving, and as such, the analytical methods used to quantify them often require adjustment and fine-tuning. With this framework and analytic support, risk managers have the decision-making tools needed when a new enterprise risk emerges.
Michelle Bradley is consulting actuary at SIGMA Actuarial Consulting Group. She can be contacted at: mb@sigmaactuary.com